นักวิจัยศูนย์ความเป็นเลิศด้านทฤษฎีและวิทยาศาสตร์การคำนวณ (TaCS-CoE) ได้สร้างอัลกอริทึมวิธีการ Conjugate Gradient (CG) ซึ่งได้รับการยกย่องอย่างสูงในการหาค่าเหมาะสมที่ดีที่สุด เนื่องจากมีคุณสมบัติทางทฤษฎีที่น่าพอใจและความสามารถที่มีประสิทธิภาพในการแก้ปัญหาที่หลากหลายในโลกแห่งความเป็นจริง ซึ่งรวมถึงการควบคุมการเคลื่อนไหวของหุ่นยนต์ การเลือกพอร์ตโฟลิโอ การกู้คืนสัญญาณ และการกู้คืนรูปภาพในการวิจัยของเรา เราได้แนะนำอัลกอริทึม CG ใหม่สองอัลกอริทึมชื่อ NSLS และ NSCD ซึ่งได้รับการพิสูจน์แล้วว่ามีประสิทธิภาพ แข็งแกร่ง และตรงไปตรงมาในการนำไปใช้
✅▶️Researchers at the Center of Excellence for Theory and Computational Science (TaCS-CoE) have created a highly acclaimed Conjugate Gradient (CG) algorithm for finding the best optimal solution. Conjugate Gradient (CG) methods are renowned for their efficiency and low memory requirements when solving optimization problems. However, certain formulations of CG methods that switch between two or more CG parameters often overlook specific values that could have been integrated. Moreover, the demonstration of the sufficient descent property in these methods usually relies on a strategy that assumes the possible exclusion of certain function values. To alleviate this assumption, this article introduces a structured Liu–Storey spectral CG method. This method extends the formulation of the spectral Fletcher conjugate descent method, enabling it to maintain fast convergence and inherit a good restart property. Therefore, the method ensures sufficient descent property holds without additional requirements and converges globally via some standard assumptions. Additionally, the method proves useful in solving robotic and image reconstruction models. Finally, it demonstrates robustness when compared to some standard algorithms.
𝗙𝗨𝗧𝗨𝗥𝗘 𝗙𝗢𝗥𝗪𝗔𝗥𝗗 ❯❯
Exploring KMUTT Research That Shapes Tomorrow
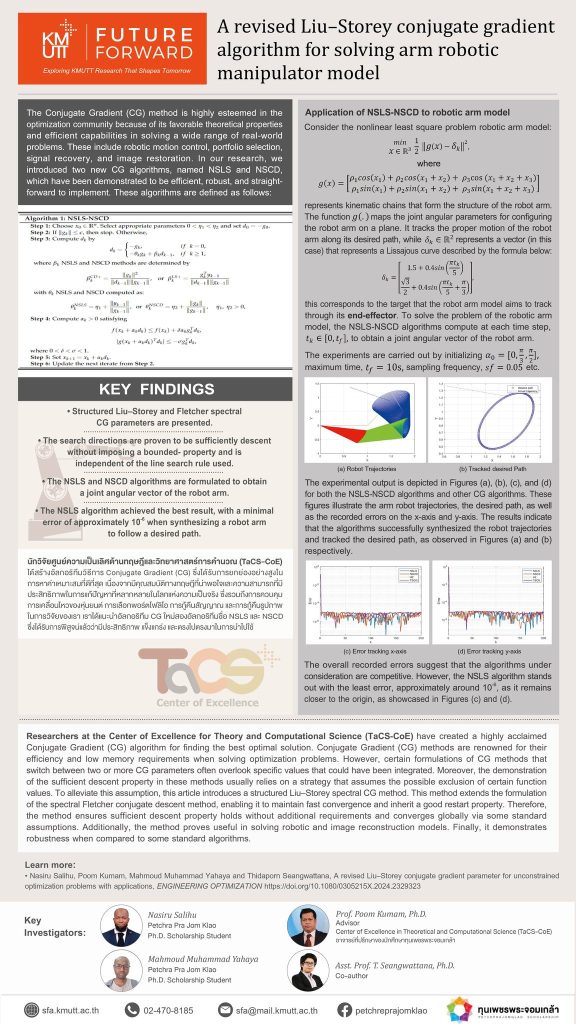